
A lightweight inference server for your machine learning models
Specially developed Open Source tool for DevOps and ML Engineers to deploy ML models in simple environments.
Simple and Fast Implementation
Easily spin up inference servers with standardized protocols that handle the scaling challenges for production use-cases.
Serve Models via REST or gRPC APIs
Deliver a swift deployment, complemented by standardized API definitions through the Open Inference Protocol
Flexible to Fit Your Requirements
The core Python inference server to used to serve ML models in Kubernetes native frameworks, making it easy to modify and extend
Smooth and Efficient Deployments
Orchestrate dependencies essential for the execution of your runtimes, ensuring a streamlined and efficient operational environment
Built With Flexibility in Mind
MLServer works for you, by giving you the flexibility to serve models according to your requirements.
- Leverage popular frameworks including scikit-Learn, XGBoost, MLlib, LightGBM, and MLflow frameworks out of the box while also allowing you to build your own custom runtimes
- Access to over 3,000 ML developers in the Seldon community to address any challenges and learn from the collective expertise within the Seldon community.
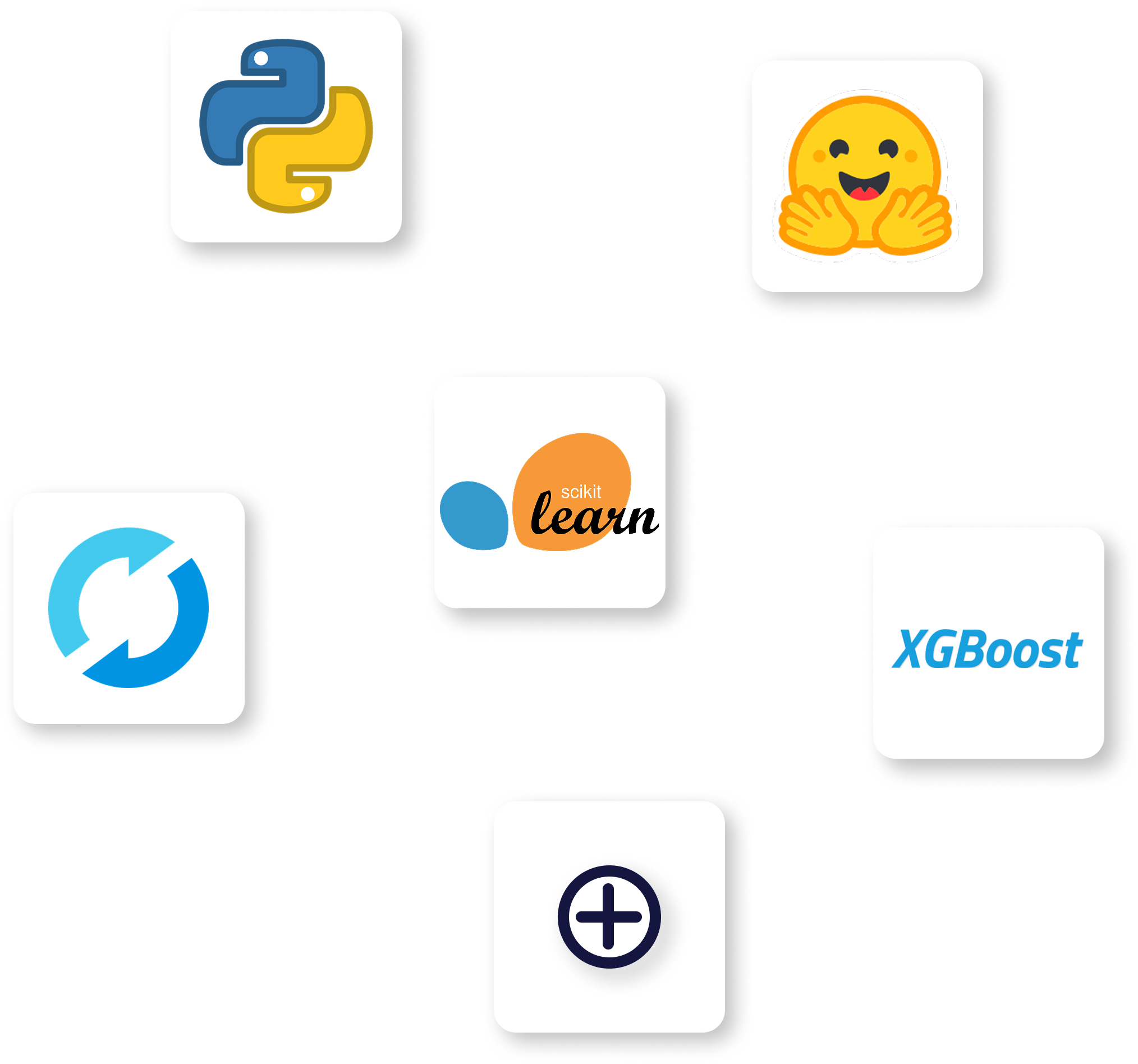
Optimize Performance
Seldon Core comes with out-of-the-box features to enhance your deployment and optimize operations and reduce latency.
Increase Speed
Reduce latency and increase throughput with parallel inference running multiple inference processes on a single server, passing requests into separately-running processes.
Optimize Resources
Improve efficiency with adaptive batching to group requests, perform predictions on the batch, and separate a batch to send responses to users to further optimize resources and improve efficiency
Infrastructure Cost Savings
Reduce cost and optimize resources with multi-model serving to run multiple models on the same server, whether it be different versions of same model or entirely different models
Stay Ahead in MLOps with our
Monthly Newsletter!
Join over 25,000 MLOps professionals with Seldon’s MLOps Monthly Newsletter—your source for industry insights, practical tips, and cutting-edge innovations to keep you informed and inspired. You can opt out anytime with just one click.
✅ Thank you! Your email has been submitted.